
For simplicity, this paper will assume that we are interested in comparing the effectiveness (or superiority) of a new treatment compared to a standard treatment, at a single point in time post-randomisation. For example, for a given power and significance level, the sample size is inversely proportional to the square of the effect size, so halving the effect size will quadruple the sample size. Sample size is critically dependent on the type of summary measure, the proposed effect size and the method of calculating the test statistic. Thus, when an investigator is designing a study to compare the outcomes of an intervention, an essential step is the calculation of sample sizes that will allow a reasonable chance (power) of detecting a pre-determined difference (effect size) in the outcome variable, when the intervention is actually effective, at a given level of significance. Sample size calculations are now mandatory for many research protocols and are required to justify the size of clinical trials in papers before they will be accepted for publication by journals. All RCTs are longitudinal, and many have a baseline, or pre-randomisation (PRE) assessment of the outcome, and one or more post-randomisation assessments of outcome (POST).įor such pre-test post-test RCT designs, using a continuous primary outcome, the sample size estimation and the analysis of the outcome can be done using one of the following methods:Īnalysis of post-randomisation treatment means (POST)Īnalysis of mean changes from pre- to post-randomisation (CHANGE)įor brevity (and following Frison and Pocock’s nomenclature ), these methods will be referred to as POST, CHANGE and ANCOVA respectively. Patient-reported outcome measures (PROMs) are now frequently used in randomised controlled trials (RCTs) as primary endpoints. There is a decline in correlation amongst more distant pairs of time points. The implications are that we can reduce the sample size in an RCT by 25% if we use an ANCOVA model, with a correlation of 0.50, for the design and analysis. There is a general consistency in the correlations between the repeated PROMs, with the majority being in the range of 0.4 to 0.6.
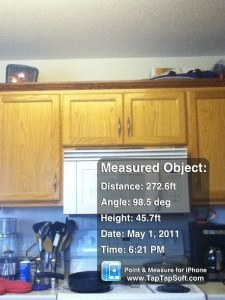
The time points for the post-randomisation follow-up assessments ranged from 7 days to 24 months 464 correlations, between baseline and follow-up, were estimated the mean correlation was 0.50 (median 0.51 standard deviation 0.15 range − 0.13 to 0.91). The 20 reviewed RCTs had sample sizes, at baseline, ranging from 49 to 2659 participants. The Pearson correlation coefficients between the baseline and repeated PROM assessments from 20 RCTs (with 7173 participants at baseline) were calculated and summarised. So what correlation (between baseline and follow-up outcomes) should be assumed and used in the sample size calculation? The aim of this paper is to estimate the correlations between baseline and follow-up PROMs in RCTs. Other parameters in the sample size estimation method being unchanged, an assumed correlation of 0.70 (between baseline and follow-up outcomes) means that we can halve the required sample size at the study design stage if we used an ANCOVA method compared to a comparison of POST treatment means method. Sample size estimation using the CHANGE and ANCOVA methods requires specification of the correlation between the baseline and follow-up measurements.
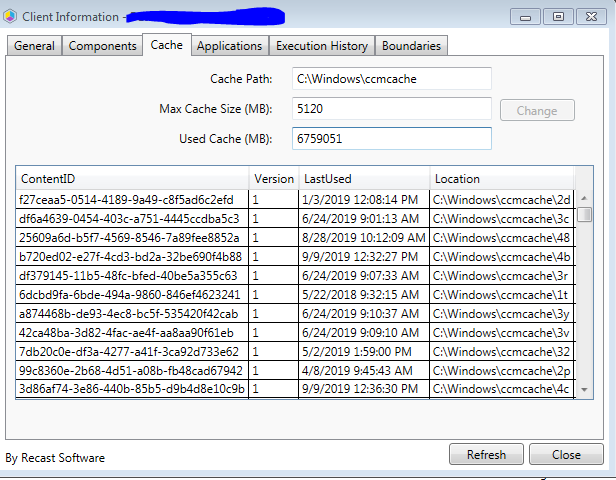
With such pre-test post-test RCT designs there are several ways of estimating the sample size and analysing the outcome data: analysis of post-randomisation treatment means (POST) analysis of mean changes from pre- to post-randomisation (CHANGE) analysis of covariance (ANCOVA). RCTs are longitudinal, and many have a baseline (PRE) assessment of the outcome and one or more post-randomisation assessments of outcome (POST).
